Startup announces technology to enable a 120-trillion-parameter model on the way to brain-scale AI.
OK, I thought I was done with preparing for HotChips, having prepared six blogs and three research papers. I was ready to take a few days off. Nonetheless, I decided to take a call from Andrew Feldman, CEO of Cerebras Systems. I have known and respected Andrew for over a decade and he always has exciting things to share. I’m so glad I took the call.
What did Andrew have to say?
Cerebras is announcing a stunning breakthrough, enabling support of AI models that are up to one hundred times larger than anything previously achieved. Using new hardware and software technologies, a single Wafer-Scale Engine based CS-2 system is capable of training an AI model with up to 120-trillion parameter. The previous record for parameter size for an AI model is from Microsoft, the MSFT-1T model, which contains (surprise) a little over 1 trillion parameters.
Now let’s put that into context; most comparisons estimate that the human brain has roughly 125 trillion synapses, which are analogous to parameters in an artificial neural network. So, an AI solution that can handle 120 trillion parameters is on the same magnitude as the human brain. And nothwithstanding whether you are a fan comparing artificial AI to the human brain, what is incontrovertible is that the Cerebras technology enables models 100 times larger anything the industry has ever seen. This is a major step forward.
In addition to increasing parameter capacity, Cerebras also is announcing technology that allows the building of very large clusters of CS-2s, up to to 192 CS-2s.
Since each CS-2 has 850,000 cores, a 192 CS-2 clusters would be a 163 million core cluster. While most companies tout the size of the cluster, and Cerebras clusters are enormous, Cerebras chose instead to focused on the ease of configuration of these large clusters. This is an interesting approach and worth examining closely, as large clusters are notoriously difficult to configure and run, oftentimes taking months. A real opportunity exists in making this simpler and easier. While diving into all this will take a longer piece, let’s take a short look at how this was accomplished.
Big Hardware for Big Models
First, let’s look at AI model growth to understand why this is important. AI models have been doubling in size (as measured by the number of parameters or weights) every 3.5 months. They have also been doubling in the amount of compute they require to train. This trend has produced 1000 times larger models in just the last two years. And these larger models require 1000 times more compute to train them. Now, we don’t believe that pace can be sustained. But clearly, the hardware needed for larger models will look very different than a commodity server with a bunch of GPUs. A different approach is needed.
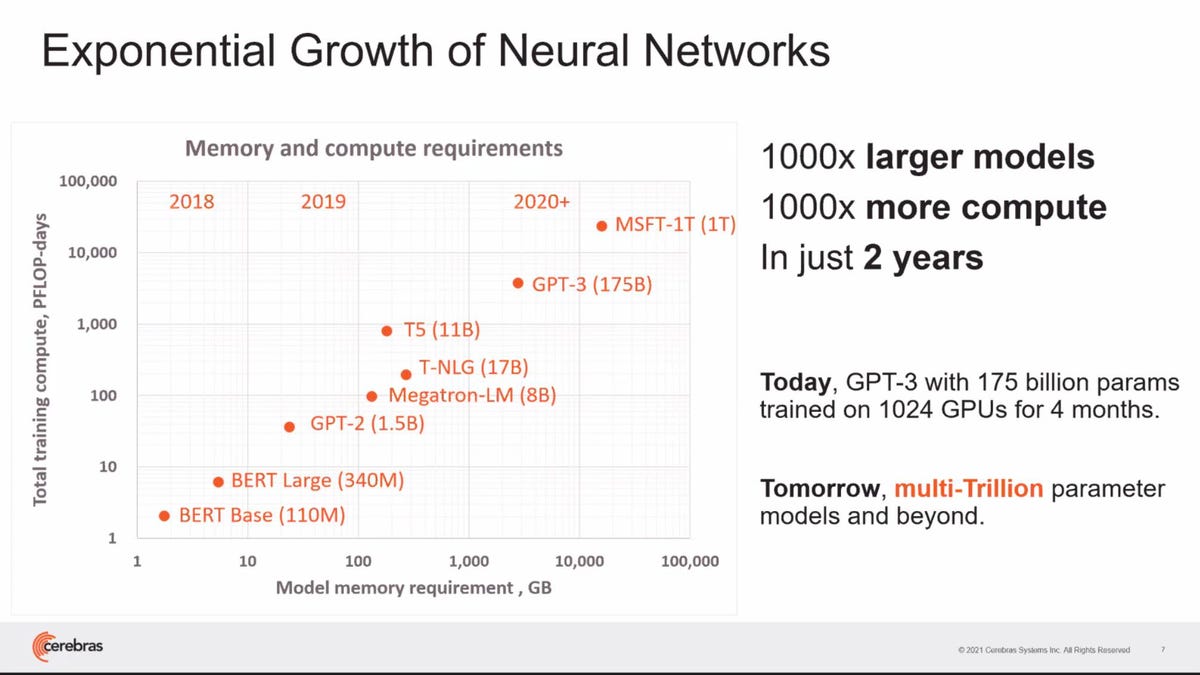
The size of neural networks has expanded by 1000 fold in the last two years. source: Cerebras Systems
NVIDIA also agrees with that assertion. Model size growth led the AI leader to announce plans for an entirely new architecture at the last GTC, using DPUs, NVIDIA’s own Grace CPU, and a next generation GPU to handle trillion-parameter models. The goal is to create enough memory bandwidth and capacity to handle them. But there is another way to skin this beast.
Cerebras announced that the CS-2 is being enhanced with new hardware and software technologies to enable massive model scaling. First is the MemoryX technology, which contains up to 2.4 PB of DRAM and flash memory to hold the massive model’s weights, along with internal computation capacity to process weight updates. Second is the new SwarmX fabric technology, which enables multi-system scaling. SwarmX essentially abstracts the CS-2 hardware to a black box to which weights are sent and gradients are received and processed. In this approach, no model parallelism is needed; every CS-2 can run the full model in its 850,000 cores and 40 GB of on-die memory where activations are stored.
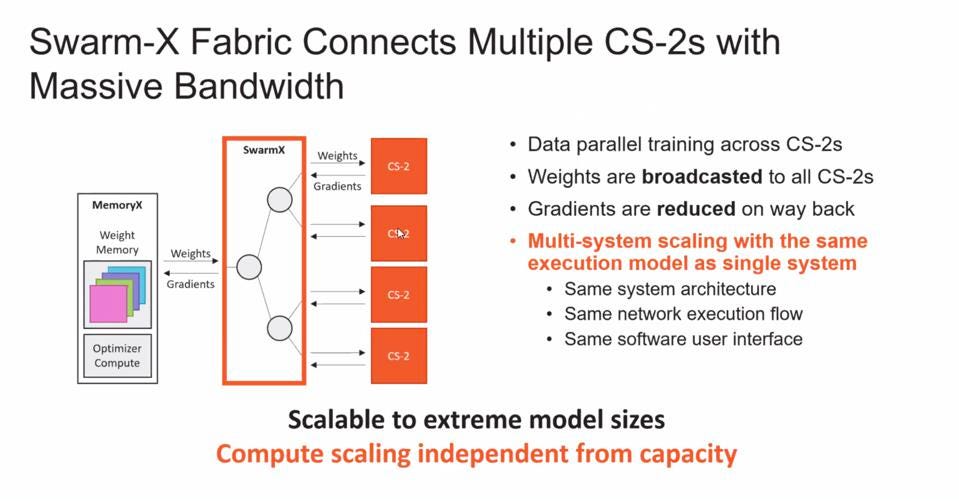
Cerebras introduced the Swarm-X Fabric which connects four CS-2 machines and specifically broadcasts weights and receives gradients from the WSE. source: Cerebras Systems
The third innovation is the software that streams weights to a cluster of CS-2 servers, retaining the programming simplicity of a single server. One of the largest challenges of using large clusters to solve AI problems is the complexity and time required to set up, configure and then optimize them for a specific neural network. The Weight Streaming execution model is so elegant in its simplicity, and it allows for a much more fundamentally straightforward distribution of work across the CS-2 clusters’ incredible compute resources. With Weight Streaming, Cerebras is attempting to remove complexities many face today around building and efficiently using enormous clusters – moving the industry forward in what I think could be a transformational journey.
Finally, Cerebras is reducing the computational complexity with extreme sparsity, not just applying brute force, demonstrating up to 90% sparsity with nearly linear benefits of speedup. Most AI chips can handle only 50% sparsity. This is enabled by having each of the 850,000 cores process only non-zero data and harvest sparsity independently.
Conclusions
Just when you thought you’ve seen everything, something new comes along and shows you just how little you really understood. Cerebras is smart. They know they cannot compete with NVIDIA on NVIDIA’s own turf. So Cerebras is changing the playing field on every dimension of AI computation for large AI’s. Will we get to human-brain levels of computational intelligence? I don’t know when, but I know we will achieve that and beyond. Perhaps then an AI could write these blogs and I could go to the mountains!