New MLPerf benchmarks lay a solid foundation; now the company needs to finish the job.
As the Cambrian Explosion reverberates across the landscape of AI chips, the benchmarking silence of many challengers stands in sharp contrast to their marketing hype. Most are vying to challenge NVIDIA, the leader in data center AI acceleration, but few have dared to take on NVIDIA GPUs in a head-t0-head benchmark using the open source MLPerf benchmarking suite. Qualcomm, the maker of the mobile Snapdragon and its AI engine, has bravely put its best foot forward, releasing its first peer-reviewed benchmarks with excellent performance and industry-leading power efficiency. We explain the results and preview what may be next for the Qualcomm Cloud AI100 platform.
Qualcomm demonstrates its prowess in performance and efficiency
While the Cloud AI100 did not outperform NVIDIA’s flagship A100 Tensor Core GPU, the Qualcomm/AMD equipped Gigabyte server nailed power efficiency in data center while the Qualcomm AI Development Kit (which uses a Snapdragon CPU) did the same in edge configurations. In all cases, the AI100 performed admirably, delivering over 3.5 times the performance of the NVIDIA T4 (albeit a two year-old platform), while consuming roughly the same amount of energy with lower latency. The Gigabyte server with the AI100 delivers performance comparable to the newest NVIDIA A30 GPU while consuming less than half the total system power, which is how MLCommons specifies that power consumption is to be measured. That means complete configurations, like the Gigabyte server with 16 cards depicted above, is far more efficient; the server’s power-hungry CPU and power supply wattage is only being amortized over a single accelerator in the benchmark.
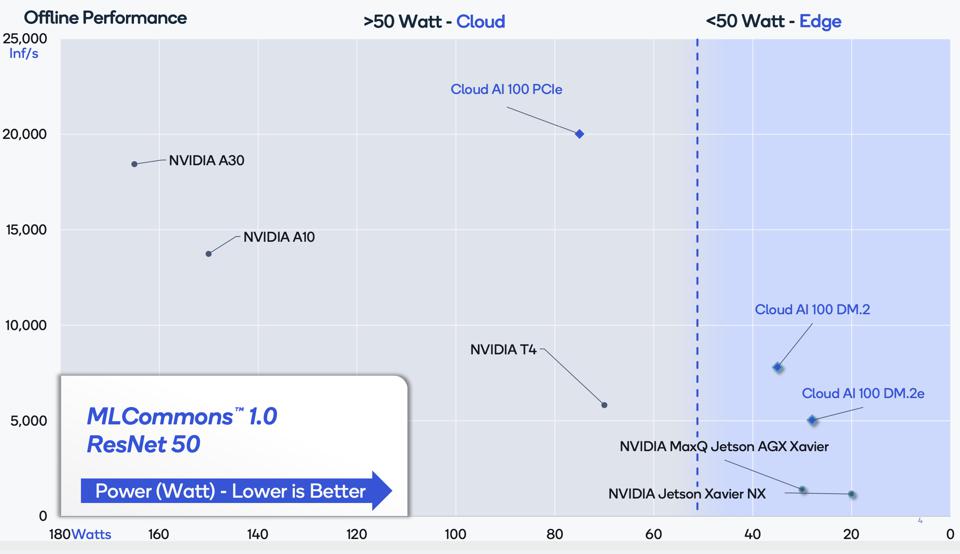
Qualcomm’s claim of industry leading efficiency seem largely validated, at least for object detection and identification. But again, these platforms are targeting different applications and deployment environments, so we remain somewhat cautious in generalizing these data points into broader assertions. For example, the NVIDIA A100 is a 250-450 watt powerhouse all by itself, and is not meant to compete with smaller, less expensive chips doing smaller jobs, while the T4 will be largely replaced with Multi-Instance fragments of a cloud-based A100 over time.
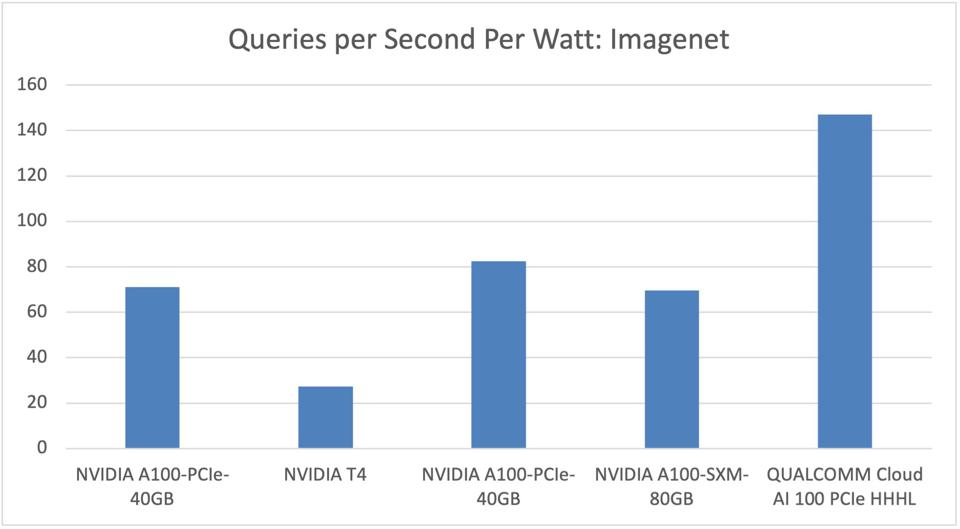
The Cloud AI100 also outperformed NVIDIA in terms of power efficiency for Imagenet, although the NVIDIA A100 delivered more absolute throughput. Cambrian-AI Research
Nonetheless, we cannot miss the opportunity to play around with a spreadsheet, and so we examine the relative performance versus latency of these contenders, this time looking at smart edge processing. The AI100 bests NVIDIA Xavier in both throughput and latency by ~5X and 3X respectively, all at lower power. Again, we must point out that Xavier has a ton of logic on chip that the AI100 does not have, but from an architectural perspective this comparison points to a significant advantage in AI model processing for Qualcomm.
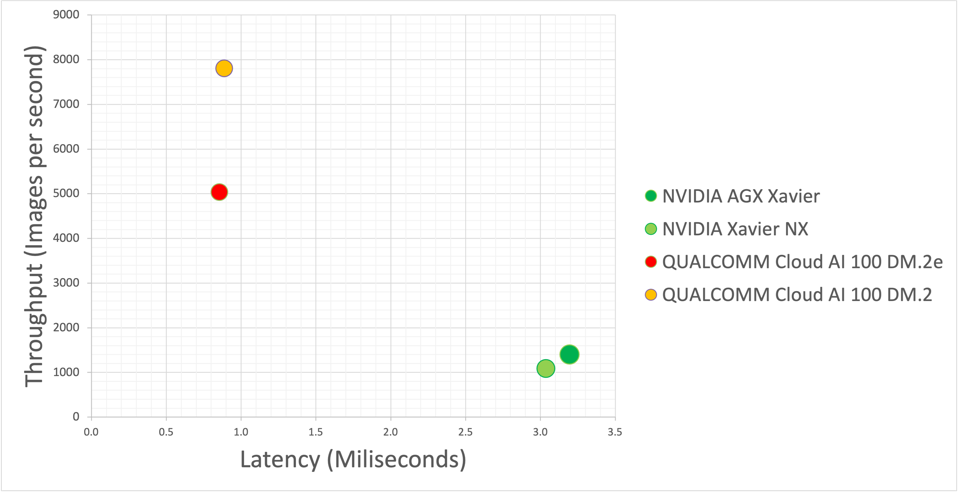
The Qualcomm AI100 has higher throughput at much lower latency than the NVIDIA Xavier part. The latter, however, provides more non-AI functionality, while the AI100 is strictly an AI engine. Cambrian-AI Research
So, what’s next for Qualcomm AI?
With these benchmarks, we believe Qualcomm has established its bona fides in data center inference processing, at least for images. The power efficiency does not come at the expense of performance, as many edge AI startups require for low power applications. But the platform is taking a long time to fully materialize as a business; we have been following it for three years now. So, now we must ask, what’s next? And what’s missing?
Looking forward, we will be anxious to understand the following.
- How well does the AI100 architecture perform in processing other AI models such as language processing and recommendation engines?
- Does the memory architecture suffice for processing larger models, or will Qualcomm need to add off-chip memory to the next generation (3- or 5-nm?) platform?
- Who are the first AI100 customers, and have any completed their evaluations? As we have speculated, the Gigabyte/AMD/Qualcomm server tells us that someone with a large data center is preparing to deploy the AI100 in volume. Otherwise Gigabyte would be unlikely to build and test a server strictly on speculation. Who is driving this demand, and who else is in evaluations? Customers are always shy; they don’t want their competitors to know what they are using that gives them a competitive advantage.
- We hope to see a TCO study or model, as power saving flow to cost of operations not acquisition. In large data center inference, TCO matters. A lot.
We remain an enthusiastic follower of Qualcomm’s entry into the data center. After the company abandoned their Centriq ARM server effort, and placed all its cloud bets on AI, this program has become a critical growth factor for the company, and initial performance and power measurements foretell a success story in the making.