IBM AI acceleration technologies are becoming so good, most AI chip companies would be better off licensing core AI tech from IBM.
Creating and using massive AI networks is quickly becoming unaffordable: Open.AI’s GPT-3 cost over $12M to train using thousands of GPU’s for weeks. But IBM believes they can address this impasse with deep research. The IBM Research AI Hardware Center was founded in May 2019 with the goal to deliver a 1000-fold improvement in AI performance and energy efficiency within ten years. In light of the three-year anniversary of the Center’s founding, we want to examine the Center’s progress and roadmap.
In short, this aggressive goal appears to be achievable given the organization’s technology and early results. Whoever says “IBM is out of the hardware business” doesn’t know about this research. Billions of dollars are being spent today to build a better AI platform, but few AI chips will be better than what IBM can provide.
Goals and Accomplishments
The IBM AI Hardware Center is undertaking four thrusts of research: Accelerated Digital AI, Analog AI, Heterogeneous Silicon Integration, and an End-User AI Testbed. The Digital project has already demonstrated the promise to reduce precision for training to four bits and for inference to two bits while maintaining acceptable accuracy. The third generation IBM AI core can deliver nearly eight-fold improvement compared to 16-bit floating point, and has already landed IBM the first IP licensee for AI, the Israeli startup NeuReality. AI HW Center IP has also shown up as AI cores in the upcoming Telum processor for IBM Z systems. The End-User AI Testbed has already delivered an Analog Composer experience to emulate in-memory compute and look to enable early access to digital reduced-precision hardware in the near future by leveraging various infrastructures including the AiMOS Supercomputer optimized for AI workloads.
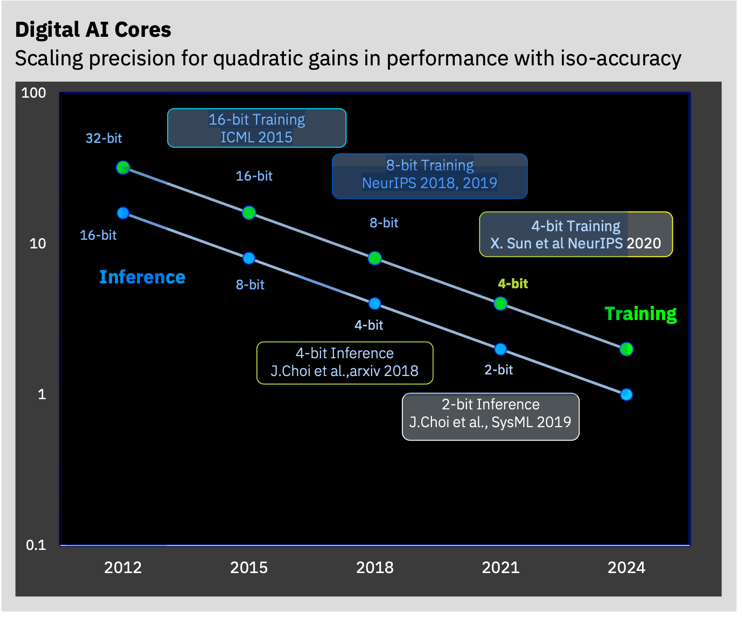
IBM Research envisions a series of advancements in AI acceleration technology. IBM Research
In addition to quantization techniques, the team is exploring how to exploit data sparsity in AI models (fine grained vs. coarse grained and random vs. structured), possibly in conjunction with extreme quantization at low precision. IBM researchers believe another 10X improvement could be had by combining these techniques over the next three years.
In the analog AI space, IBM has set itself to deliver inference technologies in the short term, and training technology in the future, processing data in-memory with goals ranging from five- to ten-fold improvement in performance per watt over the best digital architectures. While others have begun to show progress in using analog in-memory inference processing, IBM is unique in its effort to apply analog to address the massive computational and power demands of training deep neural networks.
In addition to the current analog AI devices IBM has discussed, the team is looking into various memory technologies, including PCM, RRAM, and ECRAM to achieve its goal of a 100X improvement in efficiency. Next generation AI cores with analog computing could also employ 3D silicon stacking to achieve these bandwidth improvements. All told, these additional approximate computing innovations could significantly out-perform IBM’s goal of delivering 2.5X annual improvements.
The research center is also exploring architectural designs and packaging technologies that could bear fruit. The center’s scientists are interested in improving utilization with better use of parallelism (pipeline and model), and the need to significantly improve Network-on-chip (NOC) architectures to support those techniques.
Another issue that the research teams are thinking about is model size and memory implications. Model parallelism can help here, but current designs are inadequate. Recommendation models are especially memory-hungry, which explains why most eCommerce sites still use CPU’s for these workloads that can require 10-100 Gigabytes of memory just in embedding tables. While this capacity is beyond today’s High-Bandwidth Memory device capacities, even as HBM gets larger, it drives greater cost and software complexity. Possible solutions to the memory problem could involve using 3D stacking of SRAM and DRAM with AI cores and SoCs. Tensor compression could also help here.
On the software side, IBM is investing in advancements in the IBM DeepTools compiler stack to support next-generation multi-core AI Hardware with state-of-the-art compiler optimizations. IBM intends support for multiple frameworks (Tensorflow, PyTorch) and ONNX models through a common lightweight API for runtime and offloads.
In addition to working on AI explainability, IBM is contemplating reasoning systems for Graph Neural Networks (GNNs), Logical Neural networks (LNNs), neuro-symbolic AI and other nascent AI approaches. Finally, researchers are increasingly concerned about ensuring the security of AI accelerators, especially as they are deployed in mission-critical applications. Enabling AI chips & systems to be secure E2E (through encryption), while preventing side-channel attacks on AI systems, will become critical.
Conclusions
The IBM Research AI Hardware Center has adopted an aggressive approach to AI, working not just on raw performance, but energy efficiency, model scalability, new application areas, security, and explainability. That is a broad waterfront to be sure, but the organization has already established a track record of innovations, and enabled members to build on top of a robust foundation to create greater value and innovation themselves. We expect more companies will adopt this approach and leave some of the hard work to IBM.