The Summit is full of newsworthy announcements this week! I present a look back to help kick off the event…
Today I present my annual AI industry overview at the AI Hardware Summit. This is the fourth annual event with nearly all the AI hardware vendors talking about their chips and systems. This year the focus is shifting to the latter; you can’t train massive AI models with just a chip. It takes a village. It takes a lot of memory and a highly scalable design. And damned good software.
You can view my full presentation here for those with an interest. Each of the following are covered briefly in the presentation link.
The Top 10 AI News of 2021
Its hard to pick just 10 news items from the last year, but here’s my top-of-mind list of hardware and software in AI. And don’t be surprised if I update this later this week and change my mind. The AI HW Summit has a lot of presentations to come!
- MLPerf Benchmarks
- Synopsys DSO.ai
- Sambanova, Groq, & Tenstorrent
- AWS: Inferentia (vs NVIDIA)
- Google TPU-V4
- Intel Habana Labs Gaudi
- Graphcore 2nd Generation and the IPU-Machine
- NVIDIA Hardware: DPU, A10, A30, and A40 and Grace
- Cerebras WSE-2 and Brain-Scale AI
- …. well, the founding of Cambrian-AI Research, of course! 😉
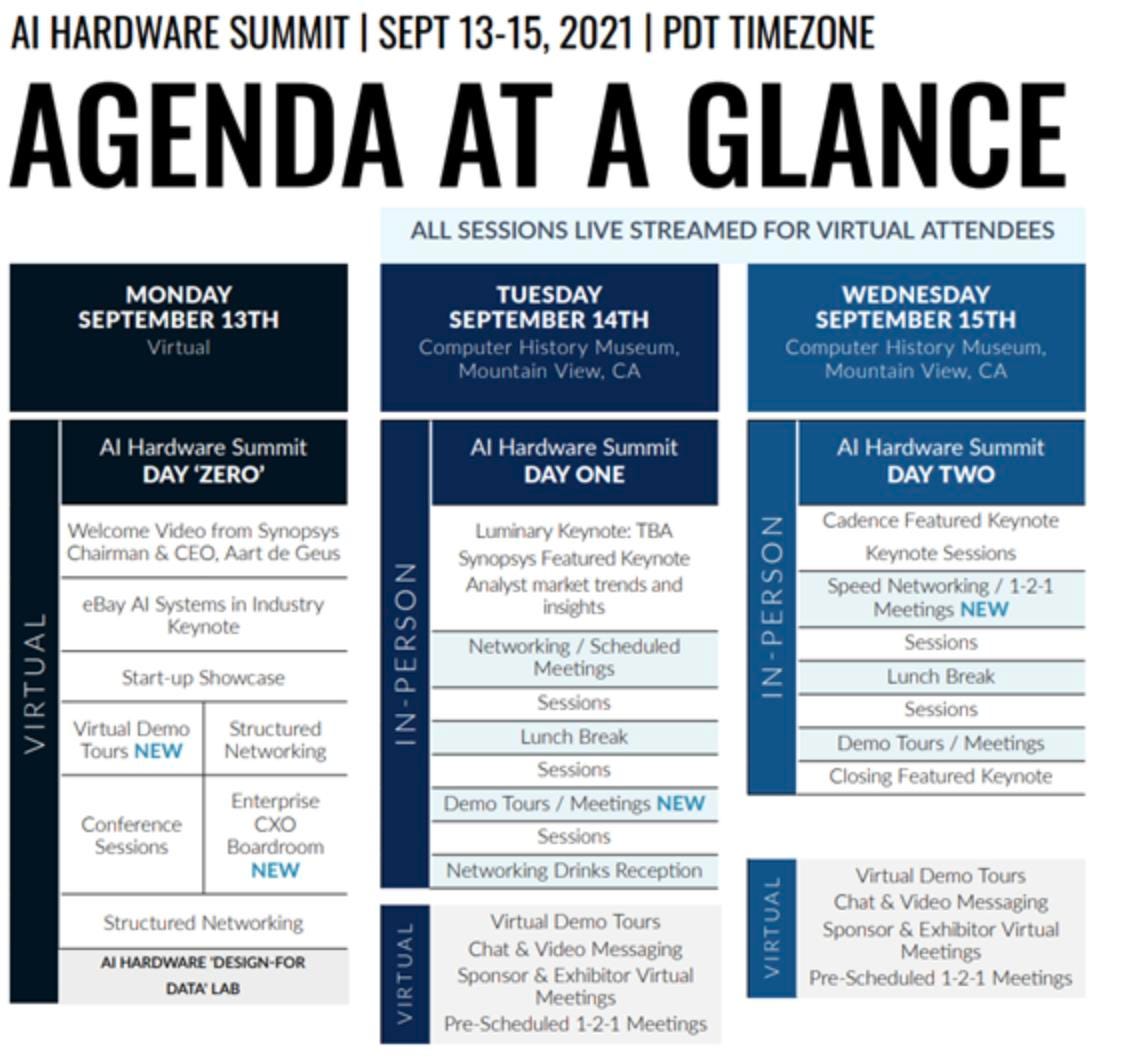
The Agenda for this years AI Hardware Summit. Kisaco Research
When it comes to large AI, its The System that matters
Since I have covered most if not all of these topics on these virtual pages on Forbes, I will focus here on the theme of the event, and the companies who are leading the way. Several vendors have come out with end-to-end AI systems, not just chips. Extremely large models (>1 trillion parameters) require rethinking the compute model, and companies like NVIDIA (Grace), SambaNova, Groq, Graphcore, and Cerebras are out in front, with Intel not far behind. Compute density alone is not sufficient in the data center of the future. It takes terabytes of memory, excellent scaling interconnects, and a software stack that not only works, but is optimized and relatively easy to use. Sounds like a supercomputer, doesn’t it? Well, it is.
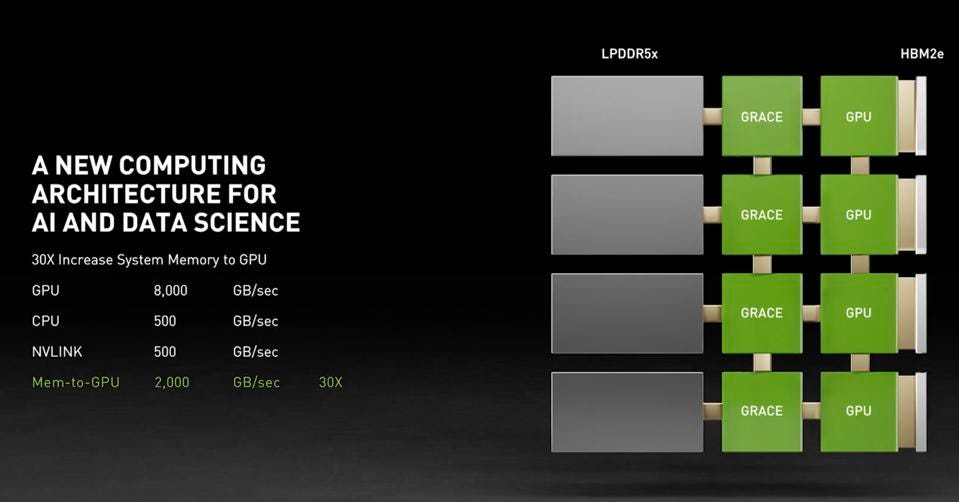
NVIDIA Grace will dramatically change the data center for high performance computing and AI. NVIDIA
Part of the problem these companies are trying to address is resolving the memory limitations of ASICs and GPUs. Big models require more memory than available on a chip, even with High Bandwidth Memory (HBM). Grace will address memory with cache-coherency across DPU, CPU, and GPU. Cerebras says they can train brain-scale models using a memory server called the MemoryX. Graphcore is solving it by complementing the on-die SRAM with a shared DRAM cache on the IPU board. And so on; each company has a unique approach, and it will take a year or two to figure out which ones work, and how large an AI model can become.
The next system-level challenge is scalability. Here NVIDIA intends to scale using NVLINK and DPUs. Graphcore’s approach is similar to Intel Habana, using 100GbE as the Level 1 networking backbone and deploying appliance-like IPU-Machines connected to disaggregated CPUs. Cerebras has a truly unique approach, scaling out with the company’s own switch, the SwarmX, and the streaming weights execution model the company unveiled at the recent Hot Chips conference.
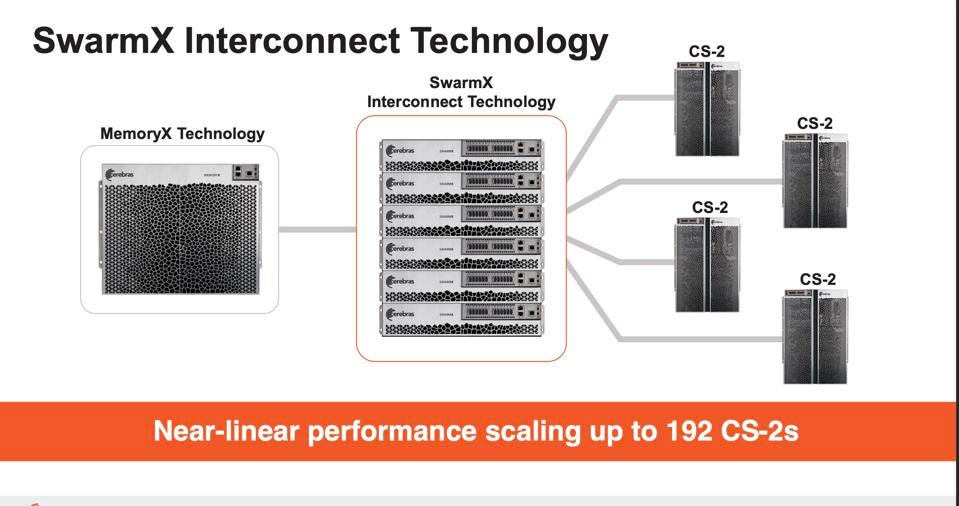
The Cerebras announcements include a new memory server and a fabric switch. Cerebras Systems
Conclusions: And the winner is…
If I choose a company based on AI business and results, the winner is NVIDIA of course. But there are a lot of cool ideas and technologies flooding into this exciting market. And Cerebras clearly leads AI HW innovation in the industry, imho. Cerebras combines the industry’s first and only massive wafer-scale engine with MemoryX memory server and SwarmX interconnect. MemoryX isn’t just a bunch of DRAM and Flash; it offloads scalar processing from the CS-2 wafers, updating the weights centrally for up to 192 CS-2 servers. Adding the Streaming Weights execution model and a super-simple deployment model puts the company in a class by itself.
Isn’t hardware fun?!